Artificial Intelligence is Exploding but Governance of the Technology Lags Miserably Behind
The costs resulting from a lack of oversight of emerging Artificial Intelligence technology can be staggering.
In both the government and private sectors, technology using artificial intelligence (AI) is everywhere. It has been incorporated across a variety of industries and has become an essential part of daily life for many. AI has an immensely powerful influence over people today; it influences many spending decisions people make, including travel, entertainment, personal purchases such as clothing, and food. While AI continues to advance rapidly in complexity, the same cannot be said for the regulations, standards, and guidelines that must be implemented alongside it. These standards and guidelines are critical to ensuring fairness, transparency, and, more importantly, accountability when AI goes wrong and causes harm. The lack of standards and guidelines is troubling, but the lack of accountability and oversight appears even more troubling.
Although there are multiple definitions of AI, the most prevalent definition refers to a machine that is capable of performing tasks that typically require human intelligence. Common examples of technology incorporating AI includes a computer that plays chess, Facebook’s newsfeed, Google’s search results, Amazon’s Echo, better known as “Alexa,” and the smartphone personal assistants, known as “Siri” or “Google.” The tasks performed by these computers are considered intelligent, meaning they typically require some higher level of cognitive functioning such as reasoning or judgment.
The processes a machine uses to learn are not really known.
While AI actually dates back to the 1950s with the famous Dartmouth Conference, AI has increased in complexity within the last ten years with recent developments in machine learning and the development of neural networks .
Machine learning, a subset of AI, enables a machine to learn without being given a specific program. Instead of traditional rules, the machine uses algorithms. However, the algorithms are not precise like mathematical algorithms that provide step-by-step instructions for solving equations. Instead, these algorithms, while mathematical, are learning algorithms that enable the computer to create models using experiential learning, much like people learn from their experiences. The computer’s experience is data-driven, and the computer “learns” by making incremental adjustments to the algorithm as it continues to receive more data.
The most common method of machine learning uses a neural network, which attempts to mimic the brain’s neurons by creating a structure of nodes, each node representing a neuron arranged in multiple layers. Minimally the network must contain an input layer, a hidden layer, and an output layer. The input layer contains multiple nodes, each representing one element of the data set. Then, each node in the hidden layer receives a weight-adjusted input from each node in the input layer and calculates an output. Finally, the output layer receives weight-adjusted input from each node in the hidden layer and then calculates the final output. This process repeats with each new set of data; the machine learns by making incremental adjustments to the weights it assigns to each input neuron to obtain an improved result.
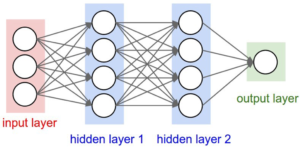
Image via http://cs231n.github.io/neural-networks-1/
This image depicts an extremely simplified explanation of the neural network. Sophisticated machine learning, referred to as deep learning, occurs when there is more than one hidden layer. Most neural networks consist of many, sometimes hundreds of hidden layers with multiple neurons in each layer. AI exploded with the advent of deep learning capability, and deep learning, in turn, created the black box problem.
AI is like an unsupervised teenager capable of causing serious harm.
The AI black box problem is the term used to describe the fact that data scientists do not know how a deep learning neural network algorithm arrived at an answer. In essence, the neural network hides the internal logic it has used. Millions of computations may be used in a neural network algorithm, and there is no current method available to explain how the network actually achieved its final result. However, scientists are currently in the process of developing new methods that will allow them to better understand the decision-making process of the algorithms within the many hidden layers of the neural network.
One idea is to use an “explanation net,” which is a second neural network that examines the first neural network and determines which neural activity is most important for determining an outcome. Another idea is to use the “observer approach,” which attempts to infer the algorithm’s behavior by using portions of the input data set to determine which input or combination of inputs has more influence in determining the result. There are other methods currently being developed; each method targets reducing or even eliminating a particular black box problem.
There are several areas of legal concern associated with AI.
The black box problem highlights only one area of legal concern related to AI. Harry Surden, a law professor at the University of Colorado, highlights many other issues, specifically, the subjective choices data scientists make in developing AI. Currently, data scientists choose the input data. What data are they including? What data are they excluding? Data scientists also choose different combinations of algorithms to create multiple models. How do they determine which algorithms to incorporate and which model is the “best” one? How do they define what makes the chosen model the best? Each of these choices has the potential to incorporate bias. What standards and guidelines are they following to make these decisions? This lack of regulation and accountability seems troubling.
What are the consequences of utilizing unregulated AI in the legal industry?
Today, legal practitioners can incorporate AI into their practices using applications capable of performing due diligence in contract review, legal research, and electronic discovery; legal analytics; document automation; intellectual property; and electronic billing. Some areas of the criminal justice system currently use AI to set bail, and determine criminal sentencing and probation decisions. These are just several areas where unregulated AI is used to make real world decisions.
Additionally, what happens when new technology makes a mistake in its decision-making that results in a devastating accident? For instance, suppose an autonomous car made a decision that resulted in a multi-injury accident. How can an attorney represent the defendant when that attorney has no explanation for what caused the accident? How is an attorney to explain in court why an unmanned drone struck a mall or a daycare facility? How can an attorney represent a bank accused of loan discrimination when there is no explanation regarding that bank’s AI-based programming and the factors that contributed to their decision? If there are no explanations for why or even how an event happened, then it would be extremely challenging to determine who is responsible for that event occurring.
More specifically, an unsettling problem arises from the use of AI-based risk assessment tools. These tools are increasingly used within the criminal justice system to help judges make bail, sentencing, and parole decisions. Essentially, the risk assessment tool, using AI, uses many factors from thousands of individuals to predict whether a particular defendant would be a high flight risk or more likely to re-offend. The goal was for these programs to reduce bias. However, stories detailing bias within these applications are frequent. Often times, unfortunately, the bias is more likely to affect disadvantaged populations negatively. In 2016, ProPublica completed a study of risk assessment tools in five states concluding that the tools more often falsely identified black defendants as more likely to commit future crime than white defendants.
Another grave concern emerges when considering rights to privacy. Major corporations, including Google, Amazon, and Facebook, utilize AI to exert influence over users. In her new book The Age of Surveillance Capitalism: The Fight for a Human Future at the New Frontier of Power, Shoshana Zuboff explains how these corporations use the data they obtain to not only target advertising to the user but to nudge or influence users’ choices. In return for allowing users free access to Google’s search engine, Facebook’s pages, or Amazon’s offerings, the corporation gains free access to the massive amounts of data resulting from the user’s search. This data, known as surplus behavior, is more valuable for the company because it can be used for purposes beyond those designed to improve the application. These giants use this data to make predictions about the user’s future behavior. For example, suppose an individual uses Google Maps on a smartphone to find a new restaurant that they want to try. Google receives all of the data related to that access, such as the destination, day, time, and the actual route taken in comparison to the route recommended. This data is likely to help Google improve Maps for all its users. However, with repeated use, Google will also have access to the type of restaurants or food that they favor, how often they eat out, whether that individual prefers going out early or later, or whether they prefer going out to eat on Tuesday or Saturday nights. Ms. Zuboff argues that the real problem is that the invasion goes beyond a mere invasion of privacy to an invasion of essential freedoms using hidden tools that modify users’ behavior.
Currently, the United States lacks any comprehensive data protection law; most law has been developed at the state level and is often limited to specific areas such as healthcare or financial information. The Obama administration proposed a Consumer Privacy Bill of Rights, which addressed how personal information could be collected and used by both the government and private companies. Unfortunately, the bill never gained momentum and was overpowered by the technology companies’ own rules. So far, the Trump administration has failed to propose any legal regulation related to data protection privacy, also allowing the technology companies to set their own rules and regulations in the interest of maximizing technology development. As a result of several groups calling for a federal privacy law, the Senate Commerce Committee continues to work on drafting such a bill, but it is unlikely to be completed or released anytime soon. In the meantime, other U.S. lawmakers continue to propose privacy bills, most of which are considerably limited in scope, and none of which are likely to become independent bills. Instead, most of the bills are offered to highlight areas that should be prioritized when crafting the comprehensive federal bill.
Governance, critical to ensure responsible AI is severely lacking.
Some advocate for excluding the use of AI from legal practice as a whole due to the lack of governance to control its use. However, others argue that throwing out existing AI because it was initially developed without standards, guidelines, and accountability is simply not an option. Too many law firms currently depend on practice management software to streamline their practices and maximize efficiency. With shrinking budgets and increased demand for services, AI allows lawyers to provide services without significant increases in costs. However, many of these applications lack a comprehensive set of standards, guidelines, and regulations to govern their use, Clearly, the need for such standards and regulations is obvious. The critical question then is who exactly is developing these and in what stage of development are they currently?
Sundar Pichai, Google CEO, posted the seven principles guiding its work in AI. Google pledges to be socially beneficial, avoid unfair bias, develop strong safety practices, be accountable, and incorporate privacy principles. Google is not alone; other tech giants such as Facebook, Amazon, Microsoft, and Apple have also developed principles and standards and even established in-house ethics boards to guide their AI development.
Internationally, the European Commission released guidelines in April 2019, but they are more general in nature, addressing seven areas of concern. The Commission recognized the importance of addressing problems with safety, privacy, transparency, non-discrimination, fairness, and accountability. The standards are designed to help legislatures draft appropriate legislation in the future. However, while the government could objectively oversee some of the standards, others are simply too abstract.
Finally, in the United States, the Institute for Electronic and Electrical Engineers (IEEE) has taken the lead in developing guidelines and standards. IEEE’s Global Initiative is designed “to ensure every technologist is educated, trained, and empowered to prioritize ethical considerations in the design and development of autonomous and intelligent systems.” Technologists include anyone involved research, design, and manufacture of AI. In furtherance of that goal, IEEE has recently released Ethically Aligned Design, First Edition, a seminal document containing specific recommendations for standards, certification requirements, regulations, and legislation of AI that align with ethical standards that are applicable around the world. For four years, several hundred leaders in academia, science, government, and corporate sectors worldwide contributed to the document by participation in one or more of its many committees.
However, the work is far from done; IEEE is still developing specific standards, and the development process is long. Each standard goes through a six-stage cycle starting with identification of a need for a specific standard, all the way to finalization and maintenance of the standard. For example, IEEE established the Algorithmic Bias Working Group in February 2017 to develop standards related to algorithmic bias.
IEEE could begin releasing standards and certification requirements for legal vendors and AI users next year.
IEEE recently announced plans to release standards for legal vendors and certification requirements for AI users as early as 2020. Once released, the vendors are likely to feel pressure to adhere to the standards because the plan also includes the ability for law firms to confirm company compliance. Additionally, certification standards for AI users will help ensure that law firms hire people who are competent in using the technology. IEEE will likely not release all of the standards simultaneously, primarily because many of them are still being developed and will only be released once finalized.
IEEE’s standards will include specific protocols, so AI engineers and designers can avoid negative bias when creating algorithms, which could lead to improved risk assessment tools should the designers choose to utilize them. The standards should also lead to improved privacy with policies that impact both the corporations that are using personal data and the individuals whose data is being used. The standards will include policies that companies are to follow in collecting and managing personal data and will apply to any of the company’s products, systems, processes, and applications that use personal data. The standards also call for individuals to have increased control over personal data through terms and conditions governing data use that individuals can tailor to their individual privacy expectations.